I presented this paper at the POM [Politics of Machines] 2024 conference in Aachen, Germany.
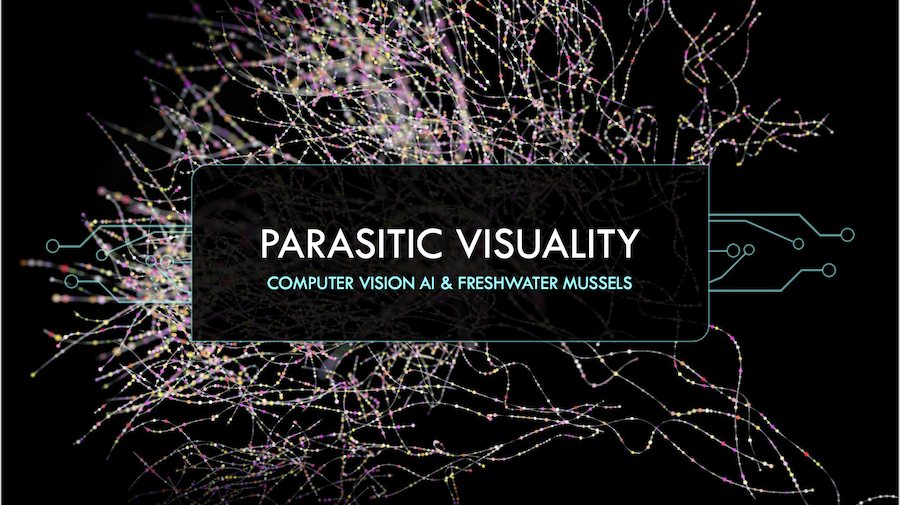
I’m going to argue that computer vision AI can be [productively] thought as a parasite, in Michel Serres’ sense – not only as a generator of informational noise, but also a producer of social and perhaps even biological parasitism, by making a comparison with another animal, the freshwater mussel, that uses vision to mediate parasitic relations. I suggest we can learn from this comparison, ways of creating more ethical and useful computer vision AIs.
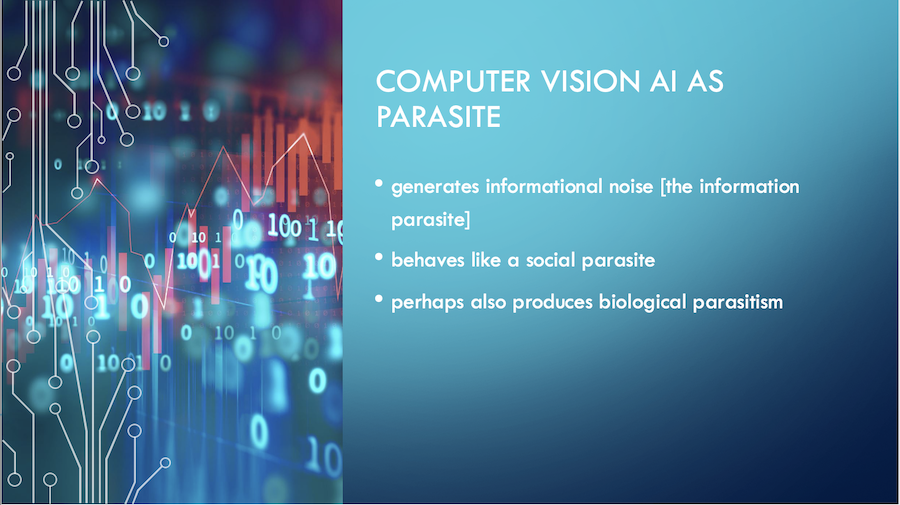
Drawing on the work of Bernard Stiegler and Justin Joque, the case can be made for AI as a form of writing. Writing, for Stiegler, is an example of what he calls a technical object, or an ‘inorganic organised being’. Following Stiegler’s technical object, we can think of AI as belonging to the same family of technical objects as writing; these are a class of semiotic technical objects that externalise memory, in which he includes mathematics.
Joque goes further, proposing that computational coding can be a form of creative writing, which makes a literary approach, after Michel Serres, particularly appropriate. Serres also offers a means of thinking ecosystemically; about interconnections between the social, biological / zoological and informational, through literary analogy.
Michel Serres argues that parasitism is the main relationship represented in narrative; where narrative is a medium for knowledge transmission in many fields and disciplines, from literature, to history, philosophy and science. He begins with the fables of La Fontaine; using them to initiate an investigation into narratives from literature, anthropology, philosophy and science.
Serres’ parasite is a relation that creates complexity in systems, but can also stop or break them. Whether biological, anthropological [social parasite] or informational [noise in communication]; the parasite displays the same behaviours: it is the noise in systems that generates complexity.
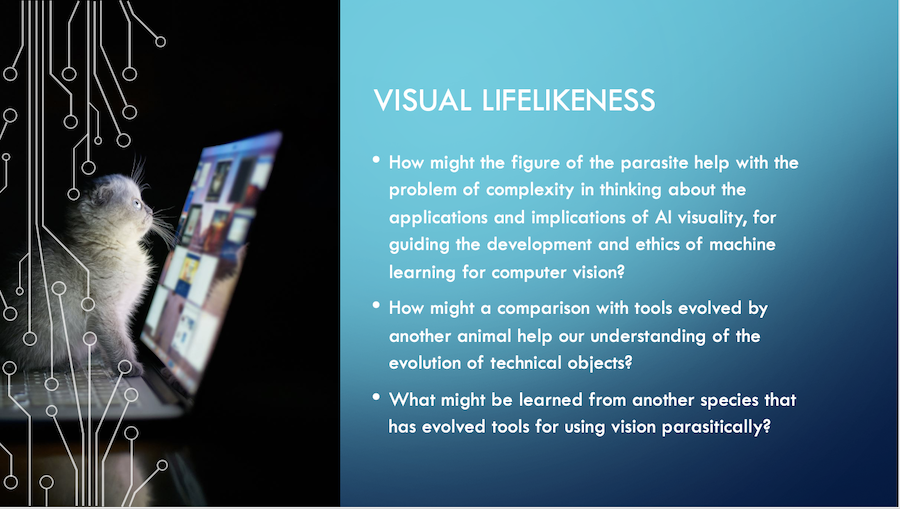
I will draw on a range of narratives, from natural history, fiction and poetry, to help think about what parasitic visuality means, through a series of metaphors, beginning with the artist-technologist as informational parasite.
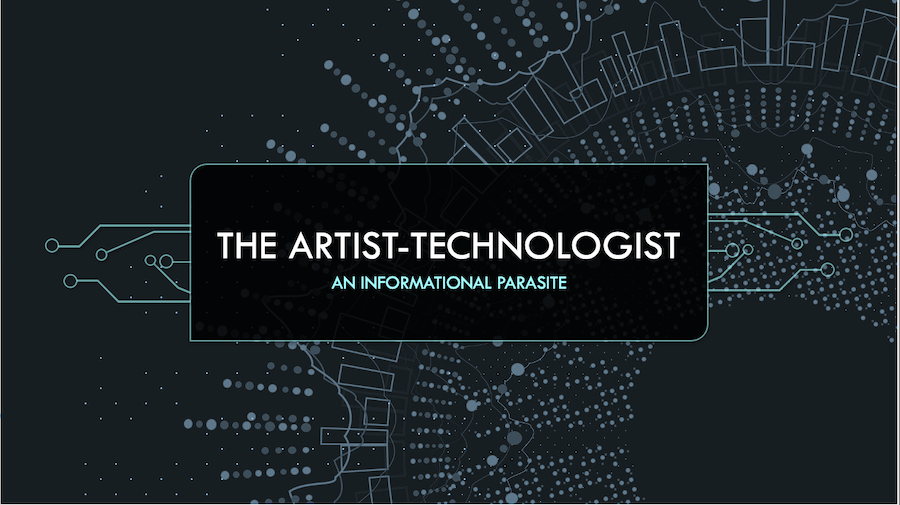
‘The Poor Artist: Or, Seven Eye-Sights and One Object: “Science in Fable”‘ (Richard Horne, 1850) was the first book ever to be described as “science fiction”, [by critic William Wilson in his 1851 review]; the author calls it a “science-fable”; it is a tale with a central idea based on then radical and new natural history research and philosophies of visual perception that suggested different people and animals see the world differently; differences between the vision of humans and other animals being greater.
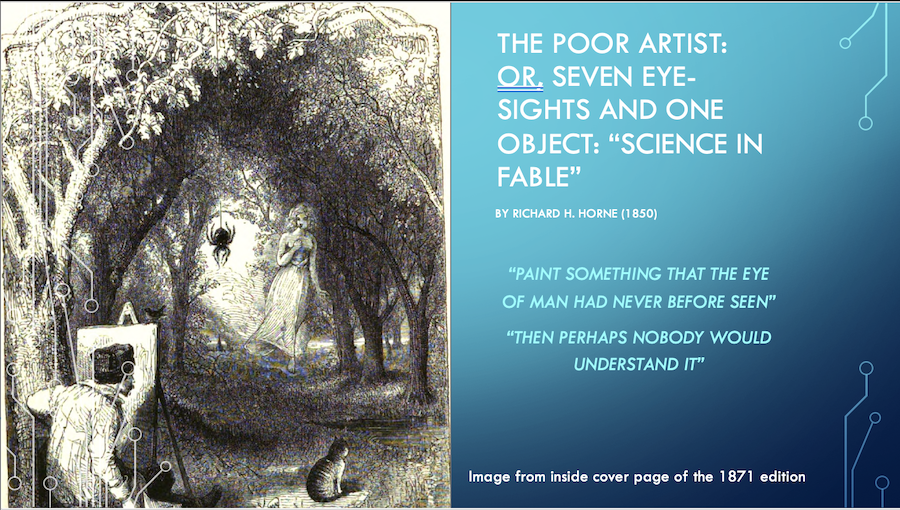
An impoverished artist needs to make his fortune so that he can marry the girl he loves. He falls asleep in a forest and, on waking, hears a strange voice addressing him. It is a bee, wanting to relay news of an alien object it has seen in the forest. The bee and five other animals – a warrior ant, a spider, a fish, a bird, a cat – who have seen alien objects in the woods each in turn describe what they have seen, and the artist paints and draws each very different vision, taking his cue from their description and adapting in response to their feedback.
Of course, it turns out that each animal has described the same object – a coin – from their very different cultural perspectives and through their different visual apparatus. The author as artist imagines how they see the same object, drawing on then-current thought about “uses of the eye” from the field of natural history – based on the eyes’ appearance, speculating what this reveals about nature of intent; the relation of ocularity to intention, use and object – what we might think as both ecologically and culturally.
The bee, for example, sees the circular coin as a hexagonal figure; the warrior ant describes the scene depicted on the coin as a grand pitched battle; the fish describes its gleam and glow, like the moon seen from underwater.
Parasiting on the vision of other animals becomes the artist’s artistic-technical innovation, with which he makes his name and his fortune, enabling him to marry the girl he loves. Critics and collectors praise these new paintings produced as a result of his encounters with other vision as strange and wonderful novelties. The painter’s audience do not know what the paintings are based on – they only perceive novelty.
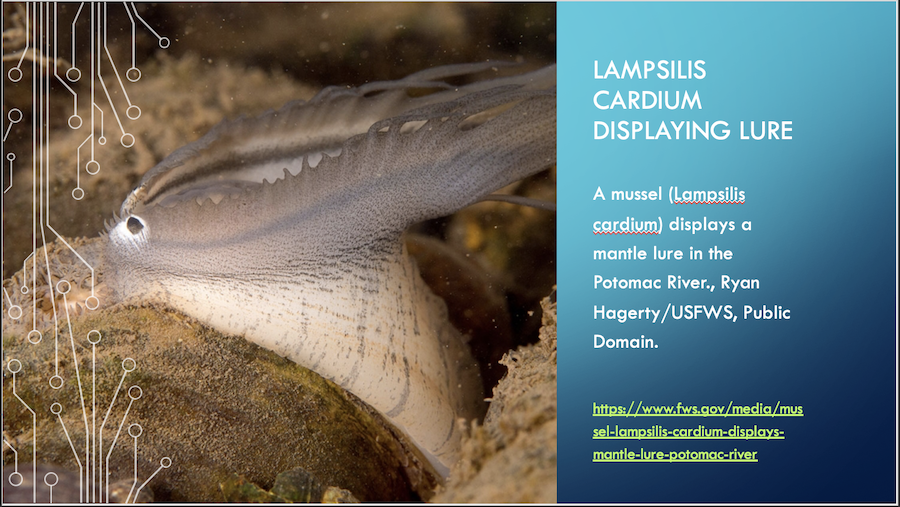
Similarly to the way in which these paintings generate communicational noise that function as a lure / attractor for critics and collectors, there are freshwater mussels like the lampsilini that create visual lures to attract fish hosts.
The ultra lifelike, visually realistic representations of small fish mussels produce to attract larger fish are often targeted to specific local species. The mussels who produce these lures cannot see them. We think of mussels as blind; they do not have eyes as such, rather they have light-sensitive spots. They parasite on fish vision to create this communicational misdirection that attracts them.
This visual parasitism can be compared to the behaviour of supervised machine learning models learning to represent objects as images for human vision, based on descriptions – labelled images – that they try to approximate, or through grouping together clusters of similar features (in unsupervised learning).
Computers, like mussels, do not have eyes; they cannot ‘see’ in the way humans do. The image to the mussel or the computer is not what the image is to the human. The AI and the mussel can produce better / more realistic seeming images apparently effortlessly and instantaneously than the human artist. Supervised AI models are trained to ‘recognise’ things, via labels that are often manually added [by ‘data janitors’], while unsupervised models like DRL try things out randomly and learn to do correct things more, which are then labelled.
Computer vision systems enable visual recognition to be automated, performed at scale and used in tasks such as object recognition, including words [Optical character recognition] and faces, pattern recognition, anomaly detection; these have a wide variety of applications from object recognition for self-driving cars and UAVs, surveillance and sports performance, to anomaly detection in medicine, manufacturing and agriculture, for example.
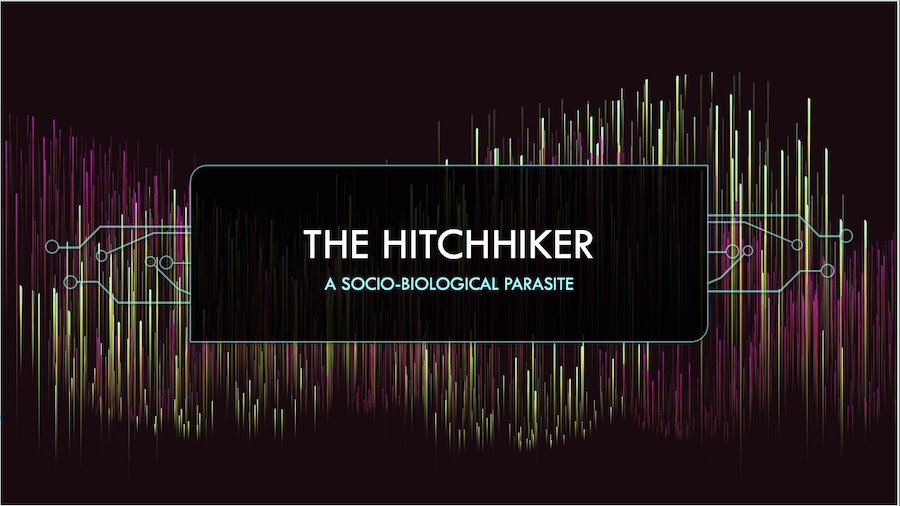
My next metaphor is the hitchhiker. In Tom Robbins’ Even Cowgirls Get the Blues (1976), which was adapted into a film directed by Gus van Sant (1993), the protagonist Sissy has an enormously oversized thumb that she uses for hitchhiking. Her thumb is a visual lure or hook that mediates this temporary, socially parasitic act.
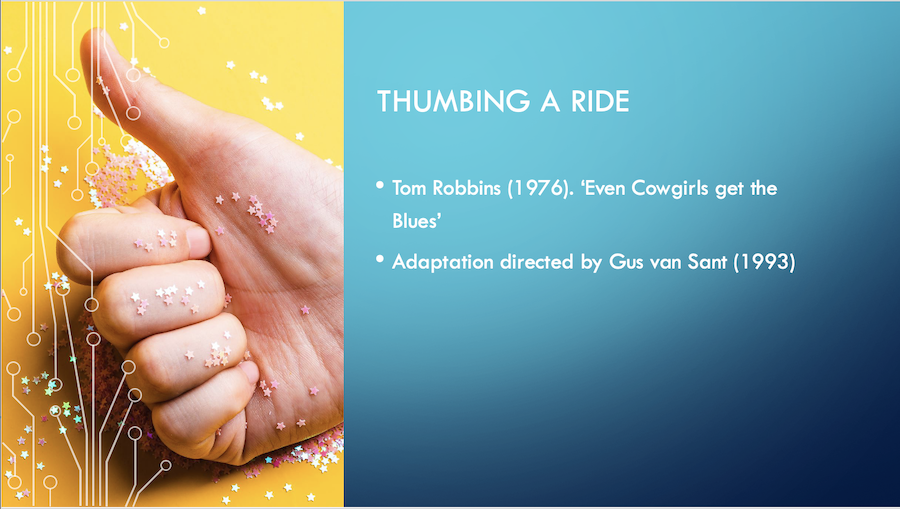
Mussel species have evolved diverse strategies for facilitating the temporary parasitism of their juveniles on fish and eel hosts. In this analogy, we can consider the lure as the mussel’s thumb. Freshwater mussels’ glochidia are, like hitchhikers, temporary parasites. They travel on fish and eels, sucking up food particles from their environment. Their impact on the host organism is usually fairly neutral resource-wise, producing only localised irritants or infections; to the extent that among zoologists there is debate as to whether they can be classified as true biological parasites. However, they certainly behave as social parasites. The juveniles themselves, called glochidia, have also evolved various somatic techniques for optimising their hitchhiking efforts. The juvenile pictured here has evolved a distinctive hook to better clasp on to fish gills.
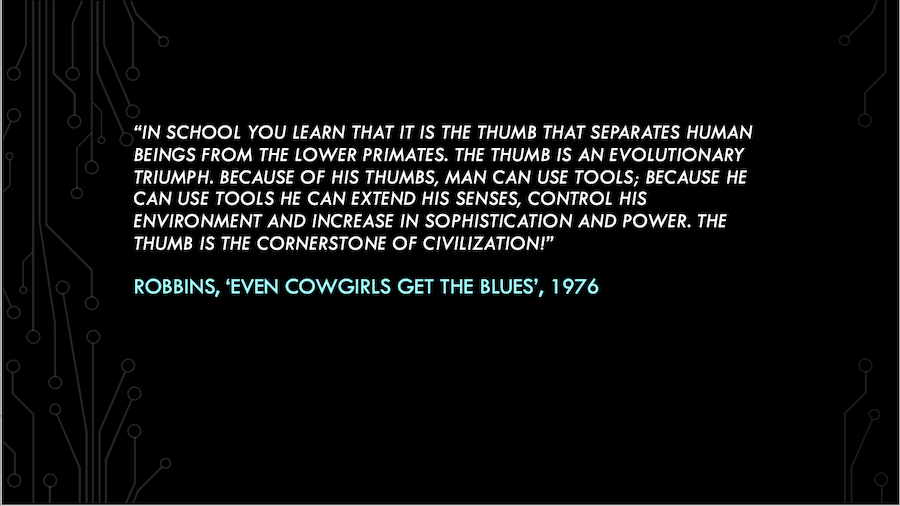
The mussel’s ‘thumb’ co-adapts to attract different local species. It affords temporary, low-impact ecological parasitism, facilitating growth and travel and new multispecies habitats. When the juvenile temporary parasites drop off the host they colonise a new area of the riverbed, establishing their own culture, ‘aquaforming’ their environment.
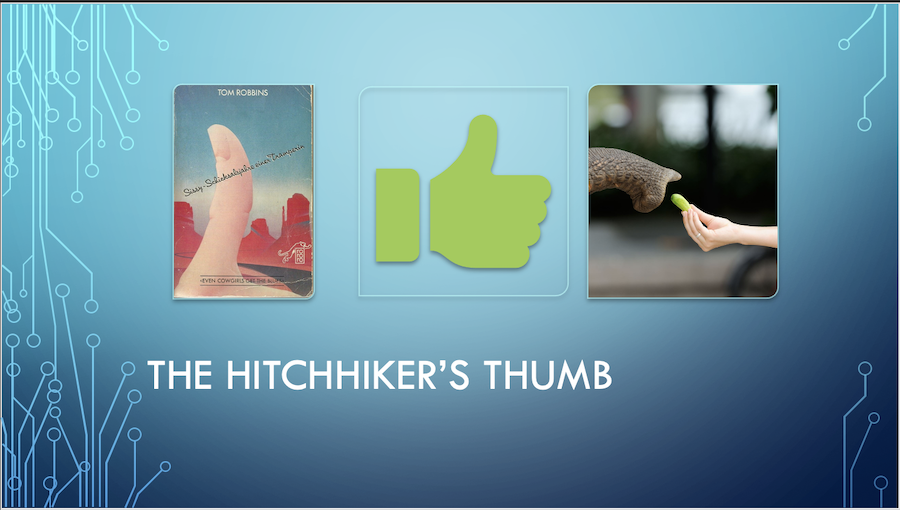
The real hitchhiker’s thumb is only a temporarily salient sign; in the dawning age of self-driving cars, the hitchhiker’s thumb is losing its meaning: a thumb becomes an emoji, a like. Its significatory shift indexes an environment shift, from a physical one to a digital one; and a corresponding move from the socio-biological realm to the socio-technical, unlike freshwater mussels that aquaform physical environments as co-evolution within them.
Is computer vision a kind of technic thumb, hitching a ride on human vision to facilitate / mediate socio-technical co-evolutions? As we will see, technical objects have been changing our uses of vision for centuries.
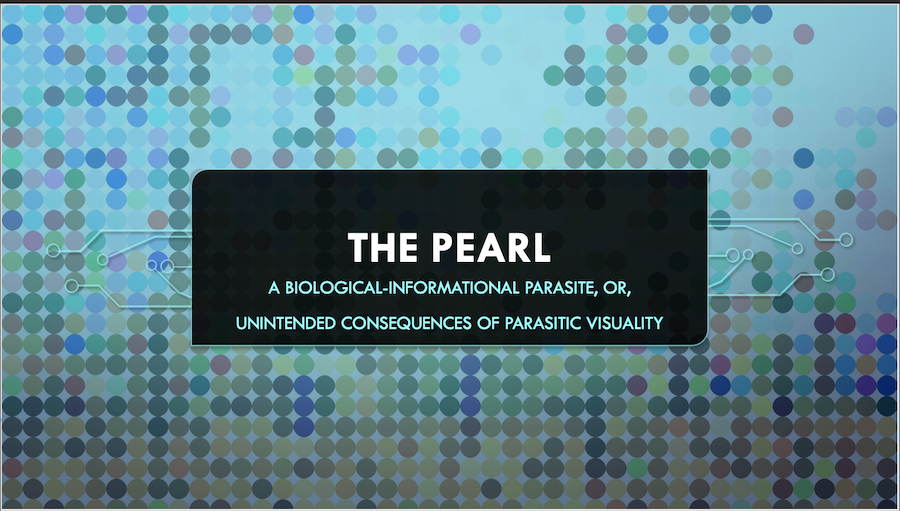
Pearls have different meanings for humans and the freshwater mussels that create them. The C14 epic poem known as Pearl is a parent’s lament for the loss of their baby daughter. It is organised around a series of pearl metaphors, to express ideas about preciousness and metaphysics; the preciousness of the daughter and her life after death in heaven; the pearl here represents much more than economic value – aesthetic and spiritual value.
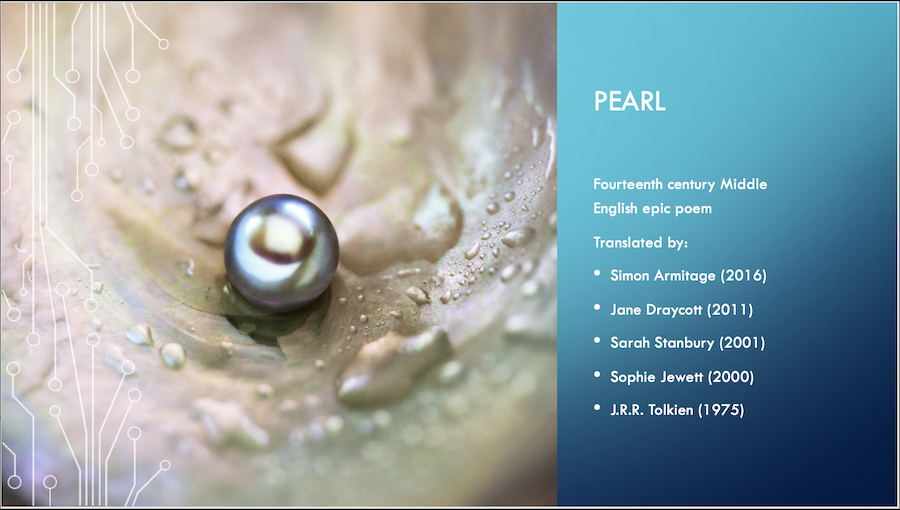
So, what are pearls to a mussel? Pearls are defence mechanisms. Mussels encase their own aspiring parasites in a sticky substance called nacre that hardens to become what we call pearl. Has the unintended evolutionary consequence of attracting another kind of parasite, a xeno-visual-parasite [evolved in a different environment]: the human.
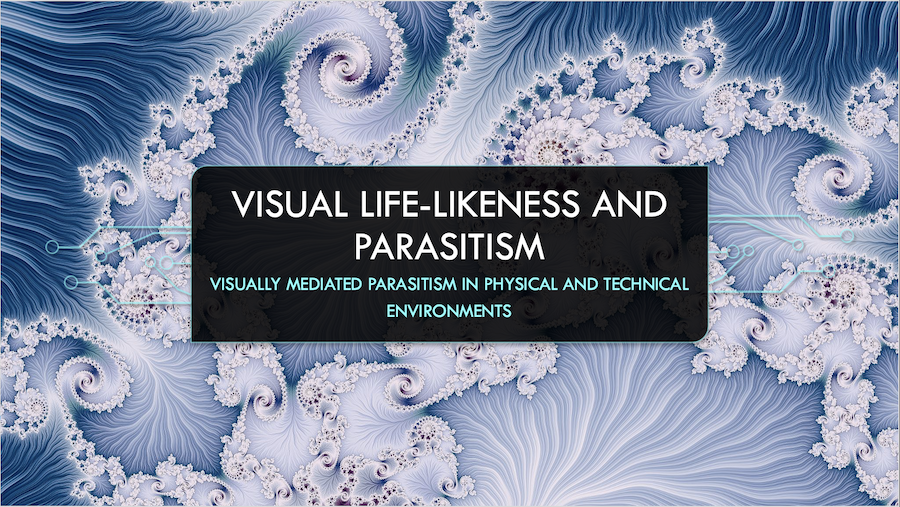
The biases inherent to the technicisation of the social are, like the human visual relationship to the pearl, xeno-parasitic, having evolved in different environments – in this case, technical environments. And they can have similarly cruel unintended consequences.
This reversal of the parasitic relation between human and computer vision AI occurs in many ways. Technical objects adapt human vision to become more suitable for what it can afford. And while the mussel aquaforms its environment, filtering and cleaning the water column and riverbed, making it a better habitat for itself and other animals, humans often terraform or adapt physical environments to become more suitable for computer vision AI.
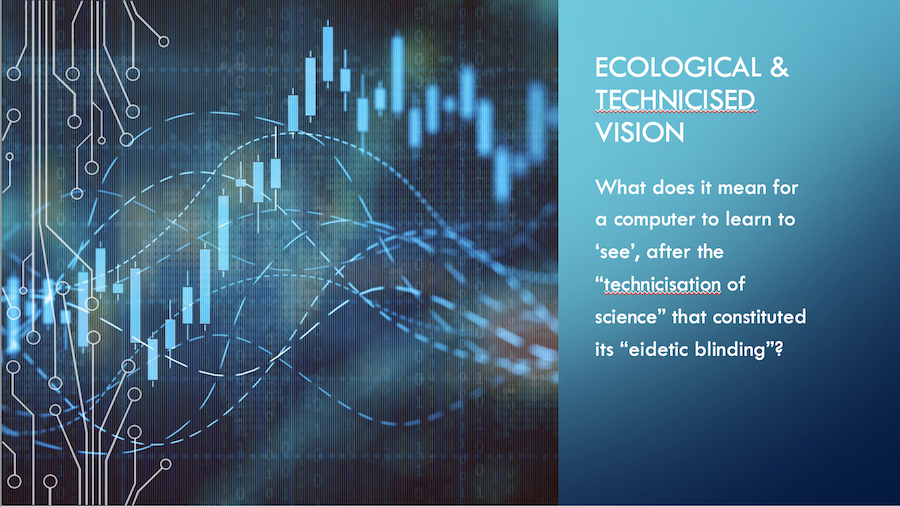
Computers and mussels do not have eyes like humans, and do not see in the way humans and other animals with eyes can; in some ways, computers and freshwater mussels are able to use vision much more sophisticatedly than humans, but they do this across and through different environments – the mussel in a watery medium and the AI in a technical one.
Mussels and AIs respond to and produce visual objects they cannot ‘see’ in the way humans can, evolving images with and for for human-like eyes. They have no visual confirmation of optimal proximation; confirmation comes through behavioural feedback of the fish or humans whose vision they are attempting to snare, captivate or mimic. Computer vision ML algorithms and mussels adapt their lures to attract different local species through a kind of trial and error process that aims to produce more of what works, or is attractive to their hosts; they do not need to know what this means for their hosts, culturally or environmentally.
Computer vision does not know about things in the same way human visual cognition or mussel evolution does; in Stieglerian-Husserlian terms, computers do not possess the [eidetic] phenomenological understanding of visual space that a human does. An AI can solve problems and perform tasks without knowing anything of the environment or context outside of the way the problem / task has been constructed for them to solve / perform.
As Stiegler points out, there are limits to the zoological analogy.
To understand this better, let’s return to the Artist-Technologist. There are obvious contradictions in Horne’s story about the artist painting other animals’ vision: the animals’ different vision would struggle to make sense of a flat, painted or drawn image on a piece of paper. These pictures, highly refined and abstracted, flattened, motionless cultural representations, only make sense in their human cultural context. Ecological vision is in constant motion, perceiving from different angles and distances; it is combined with other perceptual cues (touch, sound, smell, kinesthetics, sensorimotor).
When the first linear perspective paintings were produced, viewers had to be taught that this is how they see the world, because they did not correspond either to ecological vision or to the metaphysical representation of spaces that had been prevalent. Linear perspective paintings were new technical objects that changed the way we think we see.
Both the mussel and the AI leverage or colonise the visual perception of other animals for their own advantage; and in doing so, they change the vision they are parasiting on – they teach it to see differently. We develop new parasitic relations with our technical objects, becoming parasitic on them as we mathematise or technicise our vision and isolate it from other sensory inputs.
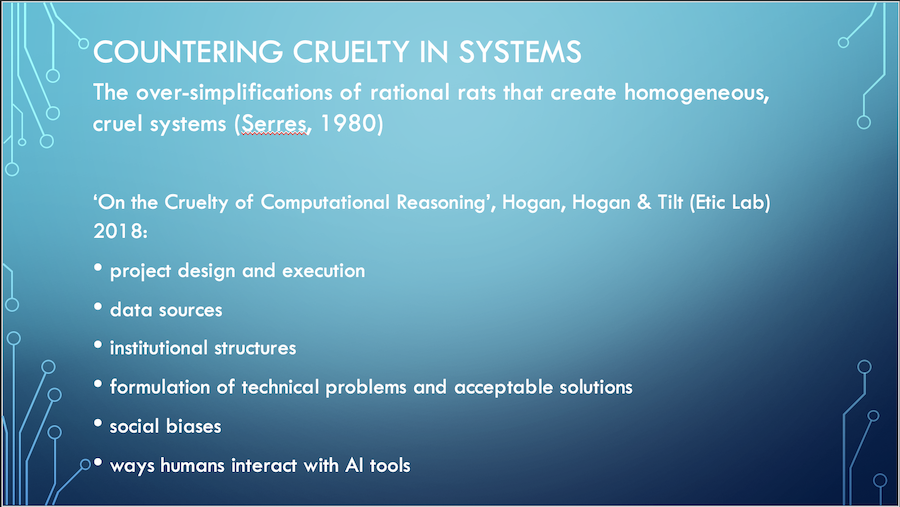
Computer vision AI extends human vision in many useful ways, for example in medicine and agriculture, but it has its limits too. It is still a young set of technologies, or tools; and, it reduces the world to statistical models.
How can we make computer vision AI more environmentally aware and more ecologically humanistic? What can be learned from mussels, from understanding that vision is not only about human sight or ocularity, and from fictional analogies, for better applications to real environments and situations? Through choice of project design and execution, data sources, institutional structures, our formulation of technical problems and acceptable solutions, and by addressing social biases and the ways users interact with AI tools, to cite a previous Etic Lab paper.
Taking a more interconnected, holistic visual model might enable more ecological mediation, that better relates to physical environments; expanding [visually-based] knowledge beyond the visual; mediating conversations with and within environments rather than mathematics being the environment. Fiction offers sophisticated devices for doing this, as a much older form of writing that has evolved to a high level of complexity.
How?
By using multiple analogue sensors in environments. Sensor technologies already used in a variety of AI smart devices, but we can extend those used for vision to an interconnected array of senses.
Problem formulation and design for divergence, using forms of ML such as Reinforcement Learning [clusters].
Producing visual forms that diverge from HD, ultra-realistic visualisations, inspired by poetics, by writing techniques and non-linear painting forms.
Narrative contextualisation, by testing in fictional environments.
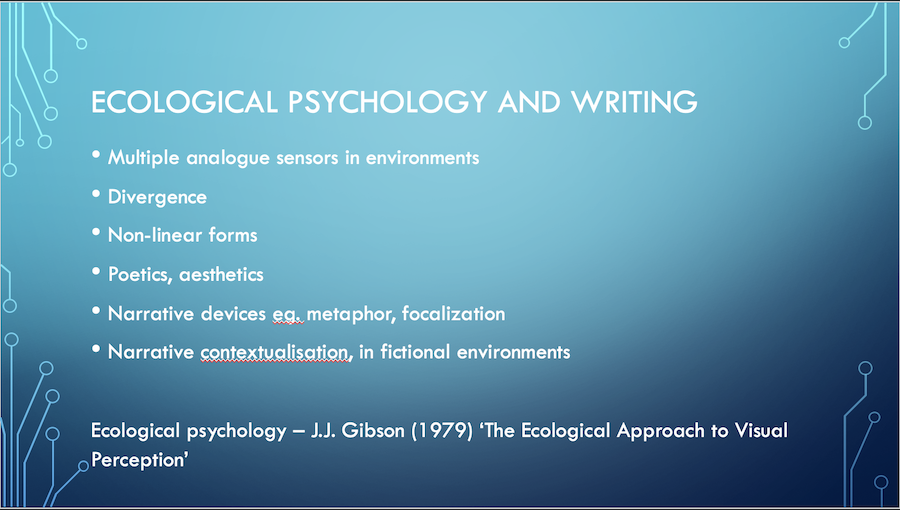
Other significant texts – Caracciolo & Kukkonen on interconnected sensory perceptions in narrative and the way we read; Tilt, Moran & Hogan on using low-fi approaches to AR, that allow the viewer-participant to supplement the provided information with their own imagination; and a novel written by an AI travelling across the US, parsing multi-sensory inputs. The freshwater mussel part is based on research I undertook in the Invertebrate Zoology department at the Smithsonian’s National Museum of Natural History, Washington DC, in collaboration with Research Zoologists John Pfeiffer and Sean Keogh.
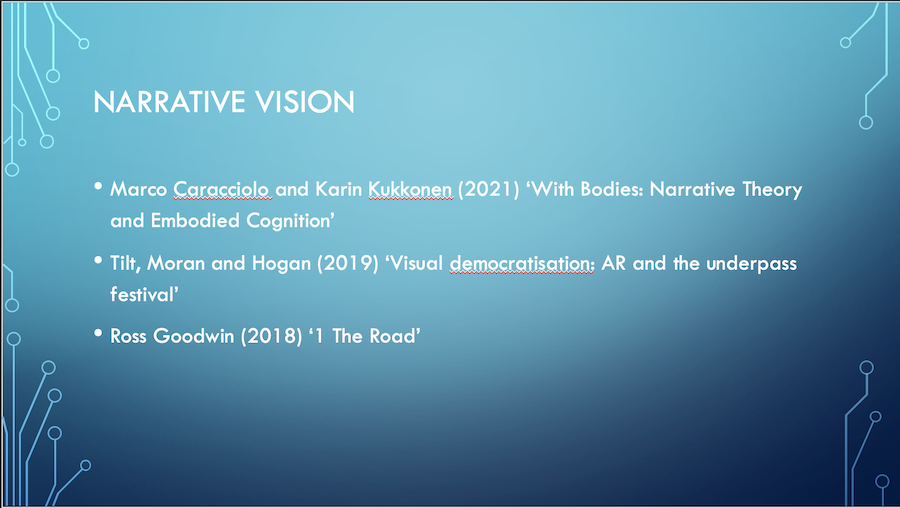